Study Level
Visiting researcher, postdoc, PhD, master, undergraduate, intern, exchange/visiting student
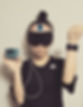
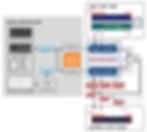
Overview
Getting enough quality sleep is a key part of a healthy lifestyle. Sleep-tracking has never been so easy with the rise of consumer wearable devices such as Fitbit and Neuroon, but do you trust what these devices tell you about your sleep? Furthermore, consumer sleep trackers are increasingly used in scientific studies to measure sleep outcomes. It is therefore of practical benefit to ensure that the sleep data collected using these devices are accurate and reliable. This study explores the accuracy issue of consumer sleep trackers both quantitatively and qualitatively. We also aim at developing computing technology that leverages the convenience of consumer sleep-tracking devices for medical-grade accuracy. This study involves the use of consumer wearable wristbands and EEG as well as medical EEG.
Research Activities
This research project contains the following two tracks
Track 1: developing new sleep staging algorithms with consumer wearables
This track may involve the following research activities:
systematic literature reviews to identify the merits and demerits of existing sleep-tracking technology
design data collection protocol and conduct longitudinal data collection experiment
retrieve and preprocess physiological data collected using a variety of wearable and mobile devices
design and evaluate new sleep staging algorithms
design sleep data visualization and perform usability evaluations
co-author research papers and give presentations in academic conferences
Track 2: human-computer interaction in sleep tracking
This track may involve the following research activities:
systematic literature reviews to identify design challenges and opportunities in sleep tracking
interviews, surveys, and co-design workshops with people with sleep disorders such as insomnia and sleep apnea
design intuitive and user-friendly sleep data visualization and perform user evaluations
design and develop new sleep-tracking technology to support good sleep hygiene
field trials to investigate the impact on behavioral change of sleep tracking technology
co-author research papers and give presentations in academic conferences
Outcomes
Upon conclusion of this research, you will gain:
experience in designing and conducting data collection experiments with human subjects
skills in data engineering and data science
experience in human-computer interaction and user-centered design
expertise in mobile health
domain knowledge in sleep science
skills in project management and technical communication
Skills and Experience
As the ideal candidate, you'll have a passion for improving sleep health and a strong background in (for track 1) machine learning and programming using Python/R or (for track 2) human computer interaction or user-centered design.
Contributors
Ms. Pataranit Sirithummarak (KUAS)
Prof. Bernd Ploderer (Queensland University of Technology, Australia)
Dr. Mario Alberto Chapa-Martell (Silver Egg Technology, Japan)
Prof. James Bailey (University of Melbourne, Australia)
Prof. Lars Kulik (University of Melbourne, Australia)
Dr. Wanyu Liu (IRCAM Centre Pompidou, France)
Dr. Yuxuan Li (University of Melbourne, Australia)
Selected Publications
Ploderer B, Rodgers S, Liang Z. (2022) What’s keeping teens up at night? Reflecting on sleep and technology habits with teens. Personal and Ubiquitous Computing.
Liang Z., Nhung H. H., Bertrand L., Cleyet-Marrel N. (2022) Context-aware sleep analysis with intraday steps and heart rate time series data from consumer activity trackers. In Proceedings of the 15th International Conference on Health Informatics (HEALTHINF 2022), Cyberspace.
Liang Z, Chapa-Martell MA. (2021) A Multi-level Classification Approach for Sleep Stage Prediction with Processed Data Derived from Consumer Wearable Activity Trackers. Frontiers in Digital Health (Section: Machine Learning and Wearable Technology in Sleep Medicine).
Liang Z. (2021) Not just a matter of accuracy: a fNIRS pilot study into discrepancy between sleep data and subjective sleep experience in quantified-self sleep tracking. In Proceedings of the 8th EAI International Conference on IoT Technologies for HealthCare (HealthyIoT 2021), Cyberspace.
Sirithummarak P, Liang Z. (2021) Investigating the effect of feature distribution shift on the performance of sleep stage classification with consumer sleep trackers. In Proceedings of the IEEE 10th Global Conference on Consumer Electronics (GCCE 2021), Kyoto, Japan.
Liang Z, Chapa-Martell MA (2021) Validation of Omron wearable blood pressure monitor HeartGuide in free-living environments. In: Ye J., O'Grady M.J., Civitarese G., Yordanova K. (eds) Wireless Mobile Communication and Healthcare. MobiHealth 2020. Lecture Notes of the Institute for Computer Sciences, Social Informatics and Telecommunications Engineering, vol 362. Springer, Cham.
Liang Z, Ploderer B. (2020) “How does Fitbit measure brainwaves”: a qualitative study into the credibility of sleep-tracking technologies. PACM on Interactive, Mobile, Wearable and Ubiquitous Technologies (IMWUT) 4(1):Article 17.
Liang Z, Chapa-Martell MA. (2020) A two-stage imbalanced learning method to sleep stage classification using consumer activity trackers. HEALTHINF 2020, Valletta, Malta.
Liang Z, Chapa-Martell MA. (2019) Accuracy of Fitbit wristbands in measuring sleep stage transitions and the effect of user-specific factors. JMIR mHealth and uHealth 7(6):e13384, DOI:10.2196/13384.
Liang Z, Chapa-Martell MA. (2019) Not all errors are created equal: influence of user characteristics on measuring errors of consumer wearable devices for sleep tracking. EAI Endorsed Transactions on Pervasive Health and Technology 18(15):e4.
Liang Z, Chapa-Martell MA. (2018) Validity of consumer activity wristbands and wearable EEG for measuring overall sleep parameters and sleep structure in free-living conditions. Journal of Healthcare Informatics Research 2 (1-2): 152-178.
Liang Z, Ploderer B, Liu W, Nagata Y, Bailey J, Kulik L, Li Y. (2016). SleepExplorer: A visualization tool to make sense of correlations between personal sleep data and contextual factors. Personal and Ubiquitous Computing 20(6): 985-1000.
Liang Z, Ploderer B. (2016) Sleep tracking in the real world: a qualitative study into barriers for improving sleep. In Proceedings of OzCHI 2016. Tasmania, Australia.
Liang Z, Nagata Y, Chapa-Martell MA, Nishimura T. (2016) Nurtuing wearable and mHealth technologies for self-care: mindset, tool set and skill set. In Proceedings of the 18th Int Conf on Networking, Applications & Services (HealthCom 2016), Munich, Germany.